Finally, after releasing the alpha and beta, today we release Docear 1.1 stable. If you have tried already one of the previous versions, there is not much news. Otherwise, read on.
Thanks to all the generous donors, our student Christoph could work on an improved PDF metadata retrieval for Docear. The new Docear 1.1 is able to extract the title of a PDF and fetch metadata from Google Scholar for that title. To do so, select a PDF in your mind-map and chose “Create or Update reference”, …
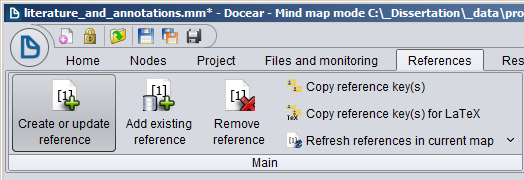
… and the following new dialog appears. The dialog shows the file name of your PDF file, and the extracted title. In the background, the extracted title is sent to Google Scholar and metadata for the first two search results are shown in the dialog. If the title was extracted incorrectly, you can manually correct it. You may also chose to use the PDF’s file name for the search. For instance, when you named your PDF already according to the title, select the radio button with the file name, and the file name is sent as search query to Google Scholar (you may also manually correct the file name before it’s sent to Google Scholar). Of course, all other options you already know are still available, such as creating a blank entry, or importing the XMP data of PDFs. Btw. Docear remembers your choice, i.e. when you select to create a blank entry, the option will be pre-selected when open that dialog the next time. It might happen, that your IP will be blocked by Google Scholar when you use the service too frequently. If this happens, a captcha should appear, and after solving it, you should be able to proceed. We did not yet test this thoroughly. Please let us know your experiences.

The precision of our metadata tool depends on two factors, A) the precision of the title extraction and B) the coverage of Google Scholar. According to a recent experiment, title extraction of our tool is around 70%. However, the final result very much depends on the format of your research articles. In my research field (i.e. recommender systems), I would say that our tool extracts the title correctly for about 90% of the articles in my personal library. In addition, almost all articles that are relevant for my research are indexed by Google Scholar (i would estimate, more than 90%). This means, for around 80% of my PDFs the correct metadata is retrieved fully automatically. Given that I provide the title manually, for even more than 90% the metadata may be retrieved. Please let us know your experience (and your research field). (more…)