Update 2019-08-12: Some numbers presented in this post were incorrect, and we updated them. For the latest full analysis, please refer to extended pre-print of Data Pruning in Recommender Systems Research: Best Practice or Malpractice?
Datasets are a key ingredient to successful research in the field of recommender systems and many other disciplines such as machine learning. However, there exists surprisingly little research on the popularity and usage of datasets in the recommender-systems community. As part of our soon-to-be-published paper on dataset pruning, we conducted a few analyses on the popularity of recommender-system datasets. We publish a slightly extended version of this analysis here in the Blog. All data is also available on GitHub.
We analyzed a total of 112 full- and short papers from the ACM Recommender Systems Conference (RecSys) 2017 and 2018. Of these 112 papers, 88 papers (79%) used offline datasets, the remaining 24 papers (21%) did not use datasets but conducted e.g. user studies or online evaluations. For the 88 papers that used offline datasets we analyzed, which datasets the researchers used.
The authors of the 88 papers used a total of 64 unique datasets, whereas we counted different variations of MovieLens (100k, 1m, ….), Amazon and Yahoo! as the same dataset.
Our analysis empirically confirms what is common wisdom in the recommender-system community already: MovieLens is the de-facto standard dataset in recommender-systems research. 40% of the full- and short papers at the ACM RecSys Conference 2017 and 2018 used the MovieLens dataset in some variations. The second most popular dataset is Amazon, which was used by 35% of all authors. Other popular datasets are shown below and include Yelp (13%), Tripadvisor (8%), Yahoo! (6%), BookCrossing (5%), Epinions (5%), and LastFM (5%). 11% of all researchers used a proprietary dataset, and 2% used a synthetic dataset.
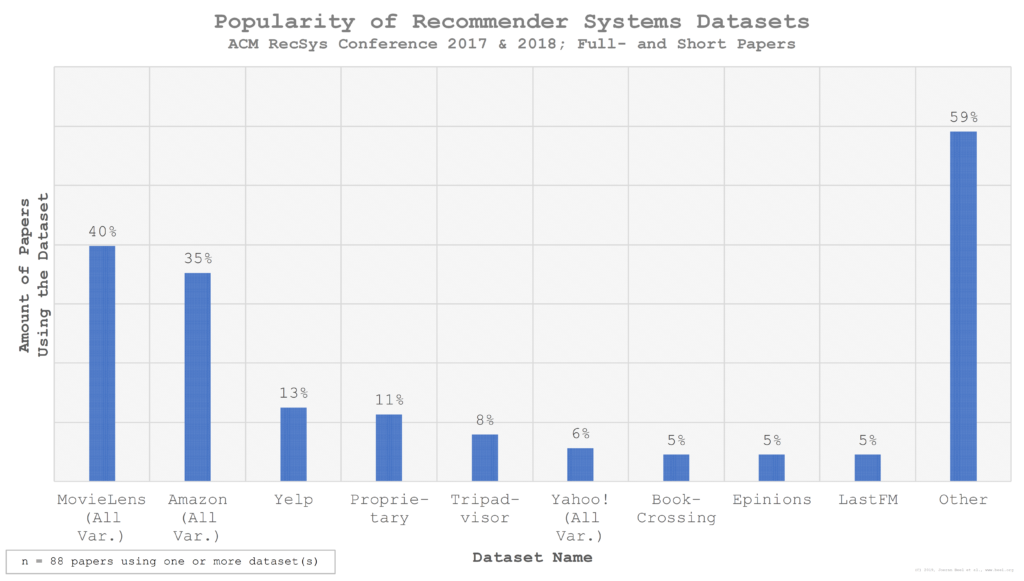
Of those 35 manuscripts having used one (or several) MovieLens datasets, the MovieLens 1m dataset was the most popular MovieLens variation (43%), i.e. of those researchers using MovieLens, 43% used the MovieLens 1m dataset (and possibly additional variations). MovieLens 20m was the second most popular variation (29%) and MovieLens 100k the third most popular variation (23%). Only one author (3%) used the MovieLens Latest, Full dataset, and no one used the MovieLens Latest, Small dataset, which is good as the MovieLens team recommends to not using the MovieLens Latest datasets for research as these datasets change over time. 11% of those researchers using MovieLens did not specify, which variation they used.
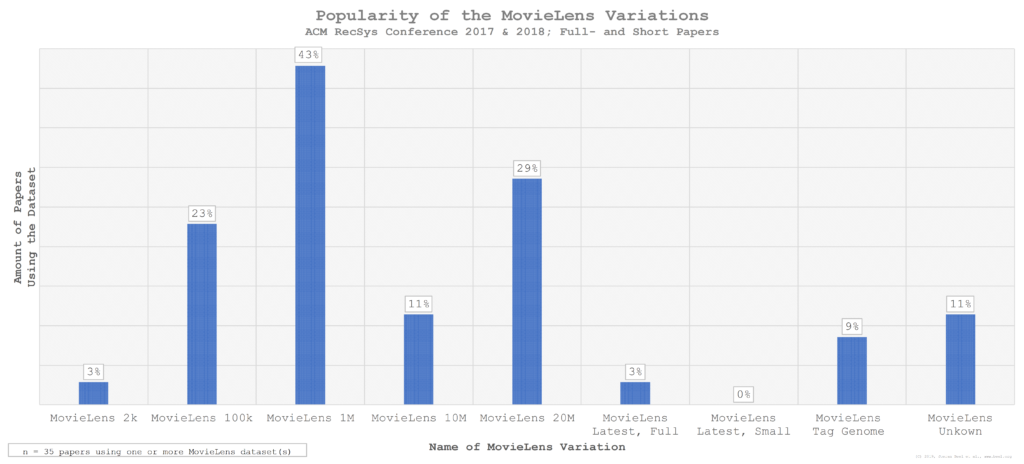
50% of the researchers conducted their research with a single dataset (if researchers used multiple variations of e.g. MovieLens, we counted this as one dataset), 31% used two datasets, and only 2% used six or more datasets (Figure 4). The highest number of datasets being used was 7. On average the researchers used 1.88 datasets.
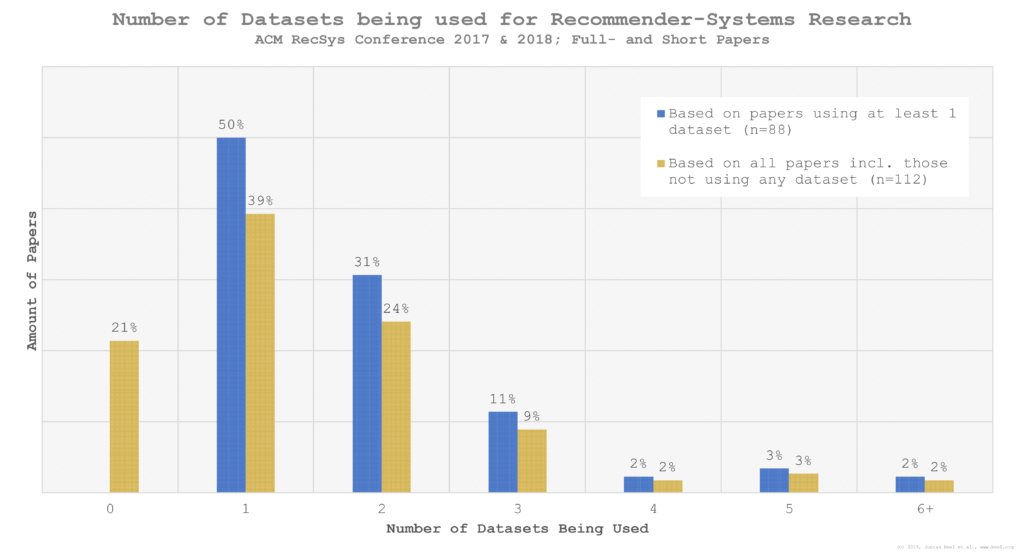
0 Comments