Abstract
We introduce Recommender-Systems.com (RS_c) as a central platform for the recommender-systems community. RS_c provides regular news on important events in the community as well as curated lists of recommender-system resources including datasets, algorithms, jobs, software, and learning materials. Based on a survey with 28 participants – mostly authors at the RecSys 2019 conference – 91% agree that RS_c could be a major contribution to the community. Participants consider it currently particularly difficult to find best practice guidelines (45%); researchers, freelancers and employers (45%); and curated lists of state-of-the-art algorithms, software, and datasets (36%). Notably, only 19% consider it (very) easy to find material relating to diversity, equality and anti-discrimination.
Original Article at ACM: https://dl.acm.org/doi/abs/10.1145/3383313.3411522
BibTeX
@InProceedings{Beel2020a,
author = {Beel, Joeran},
booktitle = {Fourteenth ACM Conference on Recommender Systems},
title = {Recommender-Systems.Com: A Central Platform for the Recommender-System Community},
doi = {10.1145/3383313.3411522},
isbn = {9781450375832},
location = {Virtual Event, Brazil},
pages = {600–603},
publisher = {Association for Computing Machinery},
series = {RecSys '20},
url = {https://doi.org/10.1145/3383313.3411522},
abstract = {We introduce Recommender-Systems.com (RS_c) as a central platform for the recommender-systems community. RS_c provides regular news on important events in the community as well as curated lists of recommender-system resources including datasets, algorithms, jobs, software, and learning materials. Based on a survey with 28 participants – mostly authors at the RecSys 2019 conference – 91% agree that RS_c could be a major contribution to the community. Participants consider it currently particularly difficult to find best practice guidelines (45%); researchers, freelancers and employers (45%); and curated lists of state-of-the-art algorithms, software, and datasets (36%). Notably, only 19% consider it (very) easy to find material relating to diversity, equality and anti-discrimination.},
address = {New York, NY, USA},
keywords = {Platform, Portal, Recommender Systems, Resources},
numpages = {4},
year = {2020},
}
Introduction
Several websites support the recommender-system community through listing recommender-system resources like datasets and software libraries or providing best practice guidelines. The probably most notable attempt to provide a comprehensive platform for the recommender systems community was RecSysWiki [6], a Wikipedia-like website to which everyone could contribute. RecSysWiki was founded in 2011 but in 2018 RecSysWiki announced that it was down [7] and until nowadays all URLs [6] lead to websites without content. Just very recently – after acceptance of the RS_c manuscript at the ACM RecSys conference, and a few days before submitting the camera-ready manuscript – RecSys Wiki seems to be online again. Other currently active services include Microsoft Recommenders with best practice guidelines and tools [1, 4]; several websites that compare recommender-system libraries [5]; and services like Google Dataset Search [2] and others [3] that facilitate the search for datasets. However, these websites focus on selected aspects of recommender systems and none, including RecSys Wiki, provides regular news on recommender systems.
Recommender-Systems.com (RS_c)
We introduce Recommender-Systems.com (RS_c) as a central platform for the recommender-system community (Figure 1). RS_c provides curated lists of recommender-system resources relating to Study & Learn (e.g. textbooks and online courses), Research & Develop (e.g. datasets and software libraries), Publish (e.g. conferences and event calendars), Meet & Discuss (e.g. Forums and Mailing lists), Best Practice Guidelines (e.g. conducting evaluations and academic writing), Funding (e.g. scholarships and travel grants) and Career (e.g. open positions and project ideas).
RS_c further provides news that are relevant to recommender-system researchers and developers. Most news is collected from third-party sources, summarized, and linked to the original source. Occasionally, we create original blog posts, especially about controversial topics to initiate discussions. Such posts, for instance, include questions like “Why is there no Recommender Systems Journal?” and “Does the RecSys community need continental chapters of the ACM RecSys Conference (e.g. a RecSysEU)”? Currently, RS_c has published 47 news items and we plan to publish around one new item every couple of days.
We tag all news and pages with the names of the corresponding authors and people being involved. For instance, the URL http://recommender-systems.com/news/tag/dietmar-jannach/ lists all posts and pages in which Prof. Dietmar Jannach is mentioned. Consequently, RS_c will be able to provide, in the long-term, a directory of people in the recommender-systems community. In addition, we aim to make the content as ´rich´ as possible, meaning we embed e.g. textbooks directly into RS_c, and provide some of the curated lists as Google Sheets to allow for easy sorting and exporting of information.
RS_c welcomes contributions from everyone in the community. However, RS_c will not primarily rely on the community to contribute content in a Wiki-style. Instead, we envision a small group of core maintainers, and occasional contributions from other community members. The core maintainers will contribute most of the content and ensure quality control. All original content is published under the free Creative Common Attribution 4.0 International (CC BY 4.0) license.
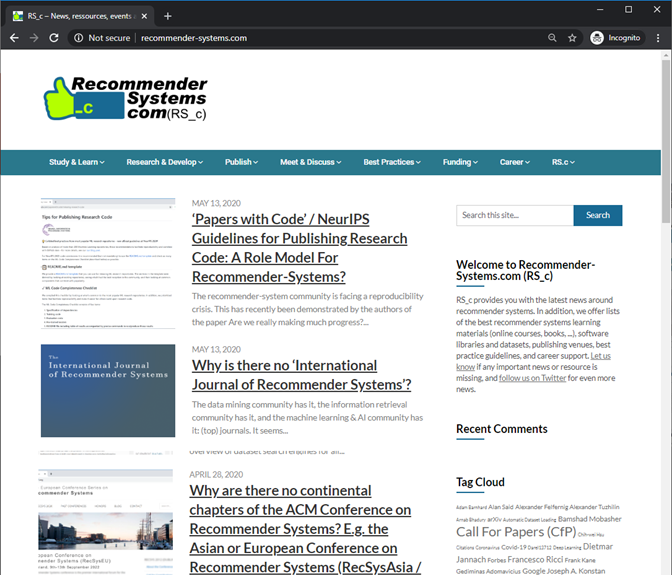
Evaluation of RS_c
We conducted a survey to explore the need for a central platform for the recommender system community, and to what extent RS_c might satisfy the need. We sent the survey to 150 randomly selected authors of the ACM RecSys Conference 2019, colleagues and students of the survey author, and promoted the survey via Twitter and LinkedIn. The survey contained a link to the RS_c website. 28 persons participated in the survey. Of the participants, 44% were female, 44% male, 11% preferred not to disclose their gender. 52% were between 31 and 40 years in age, 22% between 26 and 30 years, 15% between 21 and 25 years and 11% older than 40 years. 29% were PhD students, 21% postdoctoral researchers, 14% students (Bachelor/Master), 14% Software/RecSys/ML Engineers, and 18% professors. 67% reside in Europe, 15% in Asia, 15% in North America and 4% in Africa. 29% of the participants rated their knowledge in recommender-systems as 9 or 10 on a 10-point scale; 57% rated their knowledge in recommender systems between 6 and 8.
Our survey indicates a need for more/better curated lists of recommender-system resources, and news (Figure 2). Only few participants consider it (very) easy to find researchers, freelancers and employers (14%); career development support (18%); controversial ideas (9%); best practice guidelines (18%); news (31%); publications beyond the ACM RecSys conference (28%); curated lists of state-of-the-art algorithms, software, and datasets (27%); lists of publishing venues (32%); and places to meet and discuss (23%). Notably, only 19% consider it (very) easy to find material relating to diversity, equality and anti-discrimination. Around 20% to 45% of the participants considered it explicitly difficult to find recommender-system resources (Figure 2, bottom). Particularly difficult to find are best practice guidelines (45%); researchers, freelancers and employers (45%); and curated lists of state-of-the-art algorithms, software, and datasets (36%).
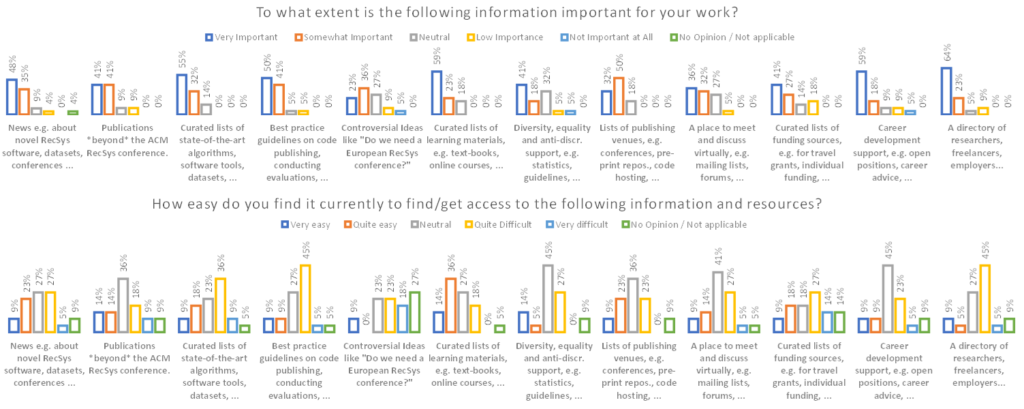
We also asked participants to what extent certain information is important for their work (Figure 2, top). Overall, participants tend to consider all the information to be (very) important. However, career-related information seems to be particularly important. 64% consider a directory of researchers, freelancers and employers to be very important, and 59% consider career development support very important. In contrast, news about recommender systems (48%), information about diversity and equality (41%), a place to meet and discuss (36%), and controversial ideas (23%) are less often considered very important.
Finally, we asked the participants about their opinion on Recommender-Systems.com. The overall feedback was highly positive. 91% (strongly) agreed that RS_c would be a major contribution to the community, under the assumption that RS_c lives up to its promise of providing curated lists of recommender-system resources and regular news (Figure 3). 68% would regularly visit the website, 64% would contribute to RS_c, and 78% would encourage their (PhD) students to visit the website. Based on the current draft of RS_c, 82% of survey participants believe that RS_c will be able to deliver its promise (Figure 4). They gave 7 or more points on a 10-point scale. The remaining 18% gave 5 and 6 points.


In summary, we are confident that RS_c will be a beneficial platform for the recommender-system community. The survey results, though small in scale, are a good first indication that many in the community will find RS_c useful and may even contribute to it themselves. Our survey also revealed interesting results on what information the community considered important and how easy/difficult it is currently to find.
Acknowledgements
This research was conducted at the ADAPT SFI Research Centre at Trinity College Dublin. The ADAPT SFI Centre for Digital Media Technology is funded by Science Foundation Ireland through the SFI Research Centres Programme and is co-funded under the European Regional Development Fund (ERDF) through Grant # 13/RC/2106. This research was further supported by the German State Ministry of Science of North-Rhine Westphalia with the NRW Rückkehrprogramm. I am also grateful for the support received by Hebatallah Mohamed, Ankit Agarwal, Utkarsh Bhardwaj, Chavvi Chandani, and Vishal Kumar.
References
[1] Argyriou, A., González-Fierro, M. and Zhang, L. 2020. Microsoft Recommenders: Best Practices for Production-Ready Recommendation Systems. Companion Proceedings of the Web Conference 2020 (2020), 50–51.
[2] Brickley, D., Burgess, M. and Noy, N. 2019. Google Dataset Search: Building a search engine for datasets in an open Web ecosystem. The World Wide Web Conference (2019), 1365–1375.
[3] Chapman, A., Simperl, E., Koesten, L., Konstantinidis, G., Ibáñez, L.-D., Kacprzak, E. and Groth, P. 2020. Dataset search: a survey. The VLDB Journal. 29, 1 (2020), 251–272.
[4] Graham, S., Min, J.-K. and Wu, T. 2019. Microsoft recommenders: tools to accelerate developing recommender systems. Proceedings of the 13th ACM Conference on Recommender Systems (2019), 542–543.
[5] Jenson, G. 2020. List of Recommender Systems. GitHub Page. https://github.com/grahamjenson/list_of_recommender_systems. (2020).
[6] Said, A. 2011. RecSysWiki. http://recsyswiki.com and http://wiki.recsys.acm.org. (2011).
[7] Said, A. 2018. RecSysWiki Twitter Account. https://twitter.com/recsyswiki. (2018).
0 Comments